Other recent blogs



Let's talk
Reach out, we'd love to hear from you!
Successful business leaders no longer solely depend on their gut feelings when making decisions. Instead, they leverage data and analytics more than ever to guide their decisions.
Business analytics is paving the way forward for today’s decision-makers. Predictive Analytics and Prescriptive Analytics are two fields that have gained significant traction.
Predictive and Predictive Analytics are related fields that can be applied to predict future trends and outcomes and swiftly change course to stay competitive and profitable.
What is Predictive Analytics?
Predictive analytics is about analyzing historical and current data to look for trends, patterns, and findings—anything and everything that can tell us something about the future regarding opportunities and challenges.
Predictive analytics is a field that combines machine learning (ML), data science, and statistical models.
The data and AI/ML teams can use techniques such as data mining, statistical analysis techniques, analytical queries, and predictive modeling to data sets to build predictive models that place a numerical value on the likelihood of a particular event happening and include what-if scenarios and risk assessment.
Forward-looking businesses use predictive analytics to guide their future decisions. Based on their findings, the leaders can build or optimize their plans to improve performance, reduce uncertainty, and deliver differentiated customer experiences.
What are the most popular Predictive Analytics techniques?
- Data mining: Data mining is the process of sifting through massive amounts of raw data to find anomalies, patterns, and correlations that can help surface insights to inform decision-making.
- Decision trees: A tree-like structure that uses questions and decisions to predict based on past data. Decision trees can help identify critical features and understand relationships between input variables and their outcomes.
- Neural networks: A machine learning algorithm that mimics the human brain to identify patterns in data. Neural networks can use multiple models, including regression, classification, clustering, and time series, to handle large amounts of data and complex relationships.
- Linear regression: A simple and interpretable technique
- Time series analysis: A method that uses previously observed data to predict future values. Time series models help predict metrics or behavior over time or when making decisions that involve uncertainty over time.
- Clustering: A technique that groups similar data points together based on criteria. However, choosing the optimal number of clusters can be difficult, and clustering models may trade off simplicity for accuracy.
Although these methods vary in approach and complexity, they all serve one common purpose: to predict future outcomes that can help businesses capitalize on what lies ahead.
Predictive analytics are mostly industry-agnostic. You can employ them to run your business smoothly, regardless of industry or market.
Common use cases where Predictive Analytics can be beneficial
Innovative organizations across several industries are increasingly investing in predictive analytics to combat the uncertainties and challenges of the future successfully. Here are some of the ways forward-looking companies can benefit from predictive analytics:
- Customer segmentation: Predictive analytics can help companies identify patterns in customer data to divide their customer base into smaller groups based on common characteristics.
- Risk management: Predictive sales analytics can help identify relationships between factors and examine risk for different scenarios, assigning a score or weight to each risk assessment.
- Predictive maintenance: Predictive analytics can be used in manufacturing to help predict when machinery might need maintenance due to wear and tear from use.
- Demand forecasting: Predictive analytics can help manufacturers provide customers with what they want at the right time.
- Churn prevention: Predictive analytics can help identify customers who are most likely to churn, allowing companies to provide better support and avoid losing them.
- Sentiment analysis: Predictive analytics and AI modeling can be used to determine customer sentiment, which can help agents gauge customer reactions and seek help when needed.
- Prescriptive analytics: Predictive analytics can predict future events and outline steps to achieve a desired outcome, producing guidelines and recommendations for action.
- Price optimization: Predictive analytics can help companies predict market conditions that impact prices and how prices impact sales.
So far, we have discussed predictive analytics and various ways they can help a business run more efficiently and profitably. However, predictive analytics are not enough. You need solid recommendations on how to act on the insights you gathered from predictive analytics. That’s where we begin to enter the territory of prescriptive analytics.
What is Prescriptive Analytics?
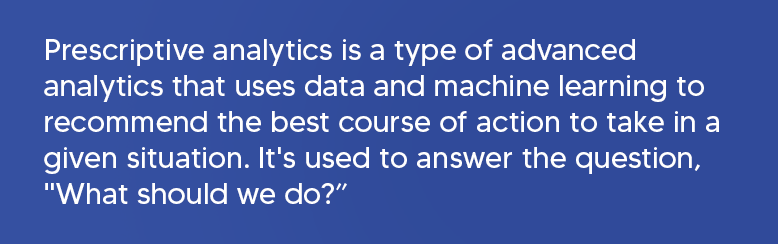
While predictive analytics helps you take a sneak peek into the future, prescriptive analytics is all about taking concrete steps toward a goal.
For this reason, many call prescriptive analytics “the future of data analytics.” After all, this kind of analysis goes beyond explanations and predictions and helps business executives decide the best course of action to drive innovation and business outcomes. It’s instrumental in driving data-informed decision-making.
Prescriptive analytics uses optimization and rules-based techniques to help businesses make decisions. Some methods used in prescriptive analytics include:
- Optimization: Techniques like linear, integer, and nonlinear programming help businesses make optimal decisions.
- Machine learning: Prescriptive analytics can use machine learning algorithms to help businesses determine the best course of action.
- Heuristics and Rules-Based Systems: These systems use predefined rules and heuristics to make decisions. They are instrumental in complex environments where decisions must be made quickly and consistently. Examples include expert systems and business rules management systems (BRMS).
Prescriptive Analytics can help businesses in a variety of ways, including:
- Energy optimization: Prescriptive analytics can help businesses increase the efficiency and dependability of their energy infrastructure by determining the best course of action for optimizing energy usage.
- Risk management: Prescriptive analytics can help businesses address risks identified by predictive analytics.
- Budget projections: Prescriptive analytics can analyze historical financial data to estimate a company's future revenue and expenses.
- Customer retention: Prescriptive analytics can analyze customer habits to identify and anticipate behavior patterns, leading to higher customer satisfaction and retention rates.
- Fraud detection: Prescriptive analytics can help businesses uncover suspicious behavior in large amounts of data, such as in a bank's system.
Predictive Analytics vs Prescriptive Analytics
Predictive and prescriptive analytics are forms of advanced business analytics that help surface insights to drive decision-making.
Predictive analytics focuses on helping business leaders look into the future and prepare accordingly. It employs complex techniques like regression analysis, data mining, and machine learning to allow business leaders to make proactive decisions and drive value across verticals.
Prescriptive analytics, however, goes a step further by forecasting future outcomes and recommending the best course of action to mitigate uncertainties while leveraging opportunities.
Predictive Analytics | Prescriptive Analytics | |
---|---|---|
What it does | Predictive modeling helps forecast future outcomes or trends based on historical data and statistical algorithms. | Suggests suitable actions or decisions to optimize outcomes based on predictive insights. |
Focus | Focuses on forecasting and understanding what might happen in the future. | Focuses on recommending the best course of action to achieve a desired outcome. |
Output | Provides predictions, probabilities, or forecasts. | Provides actionable recommendations or decision options. |
Example | Predicting customer churn, sales forecasting, demand prediction. | Recommending optimal pricing strategies, resource allocation, treatment plans. |
Time frame | Looks into the future based on historical data. | Looks into the future and provides actionable steps to influence outcomes. |
Decision making | Aids decision-making by providing insights into potential future scenarios. | Guides decision-making by recommending the best actions to achieve desired outcomes. |
Parting thoughts
In an era where data is hailed as the new oil, the transition from instinct-driven to data-driven decision-making marks a profound shift in how businesses operate.
Predictive and prescriptive analytics are at the forefront of this transformation, empowering leaders to foresee future trends and craft strategies that achieve desired outcomes.
Predictive analytics equips businesses with a crystal ball, offering glimpses into the future. However, predicting the future isn't enough in today's competitive landscape.
This is where prescriptive analytics takes the baton, transforming insights into actionable strategies that drive tangible results. It's akin to having a seasoned guide who anticipates the weather and charts the safest and most profitable course through the storm.
At Kellton, we help our clients unleash the full potential of data with our world-class data engineering solutions. Our team ensures seamless integration of predictive and prescriptive analytics into their operations, delivering the insights and recommendations necessary to achieve their strategic objectives and excel in a competitive landscape.
To embark on this exciting journey, contact Team Kellton!